The term “AI” has been used in computing since the 1950s, but most people outside the industry didn’t start talking about it until late 2022. This is because recent advances in machine learning have given rise to great advances that are beginning to have a profound impact on almost every aspect of our lives. We’re here to help you break down some of the buzzwords so you can better understand AI terms and take part in the global conversation.
1. Artificial intelligence(AI)
Artificial intelligence is basically an intelligent computer system that can imitate humans in some ways, such as understanding what people say, making decisions, translating between languages, and analyzing whether it is wrong or right. good and even learn from experience. It is artificial in the sense that it is man who uses technology to create his intelligence. Sometimes people say the AI system you have a Digital Brain, but they are not the body machine or robots – they are the program running on the computer.
They work by applying many data from algorithms, which is a method of instruction, to create forms of people who can repeat activities at time. Sometimes people interact directly with the AI system — like asking Bing Chat for help — but more often, AI happens in the background around us, suggesting words as we go as we type. books, suggest songs and playlists and give away. Other important information depends on our preferences.
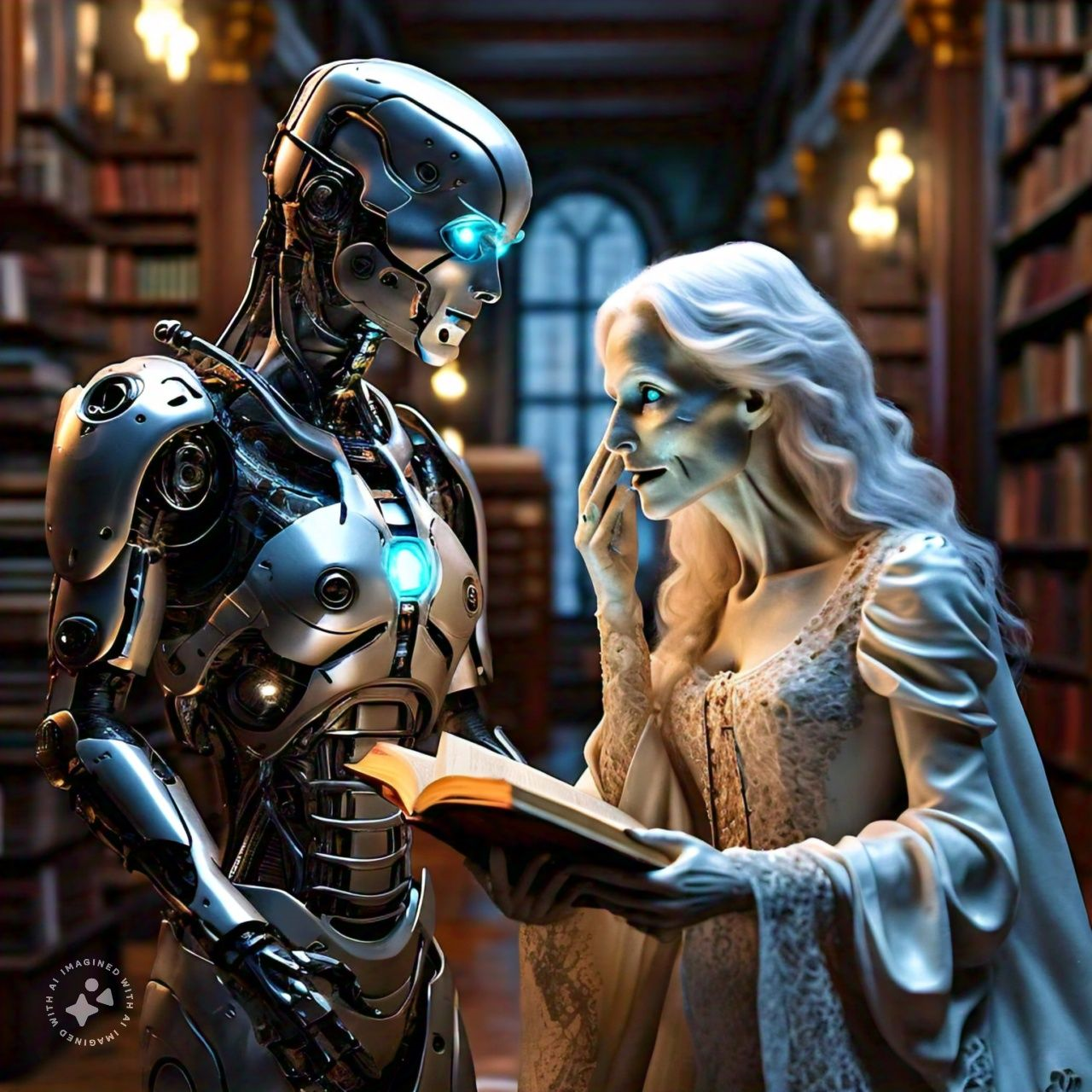
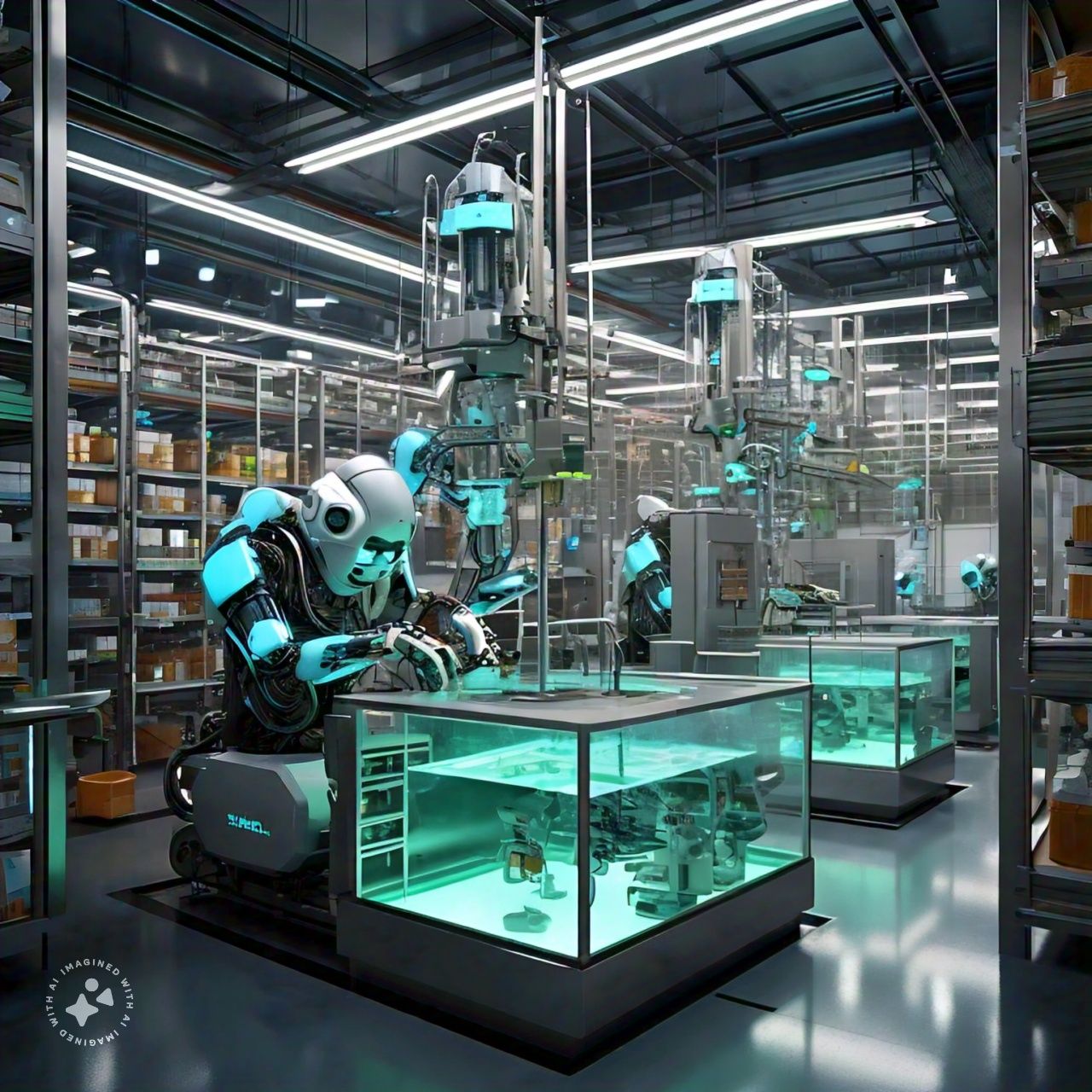
2. Machine learning(ML)
If the goal is artificial intelligence, machine learning is the way to get there. It is a field of computer science, under the umbrella of artificial intelligence, in which people teach a computer system how to do something by training it to identify patterns and make predictions based on them.
The data is passed through algorithms over and over, with different input and feedback each time to help the system learn and improve during the training process, like practicing piano scales 10 million times so you can sight-read the scores on the computer. It is especially useful with problems that would otherwise be difficult or impossible to solve using traditional programming techniques, such as image recognition and language translation.
It requires a huge amount of data, and it’s something we’ve only been able to take advantage of in recent years as more and more information has been digitized and computer hardware has become faster, smaller, more powerful, and more capable. to process all that information. That’s why great language models that use machine learning, like Bing Chat and ChatGPT, suddenly appeared on the scene.
3. Large language Models(LLM)
Large language models, or LLMs, use machine learning techniques to help them process language so they can mimic the way humans communicate. They are based on neural networks, or NNs, which are computer systems inspired by the human brain, something like a set of nodes and connections that simulate neurons and synapses. They are trained with large amounts of text to learn patterns and relationships in language that help them use human words. Its problem-solving skills can be used to translate languages, answer questions in the form of a chatbot, summarize texts, and even write stories, poems, and computer code. They don’t have thoughts or feelings, but sometimes it seems like they do, because they have learned patterns that help them respond as humans would. Developers often refine them through a process called human feedback reinforcement learning (RLHF) to help them sound more conversational.
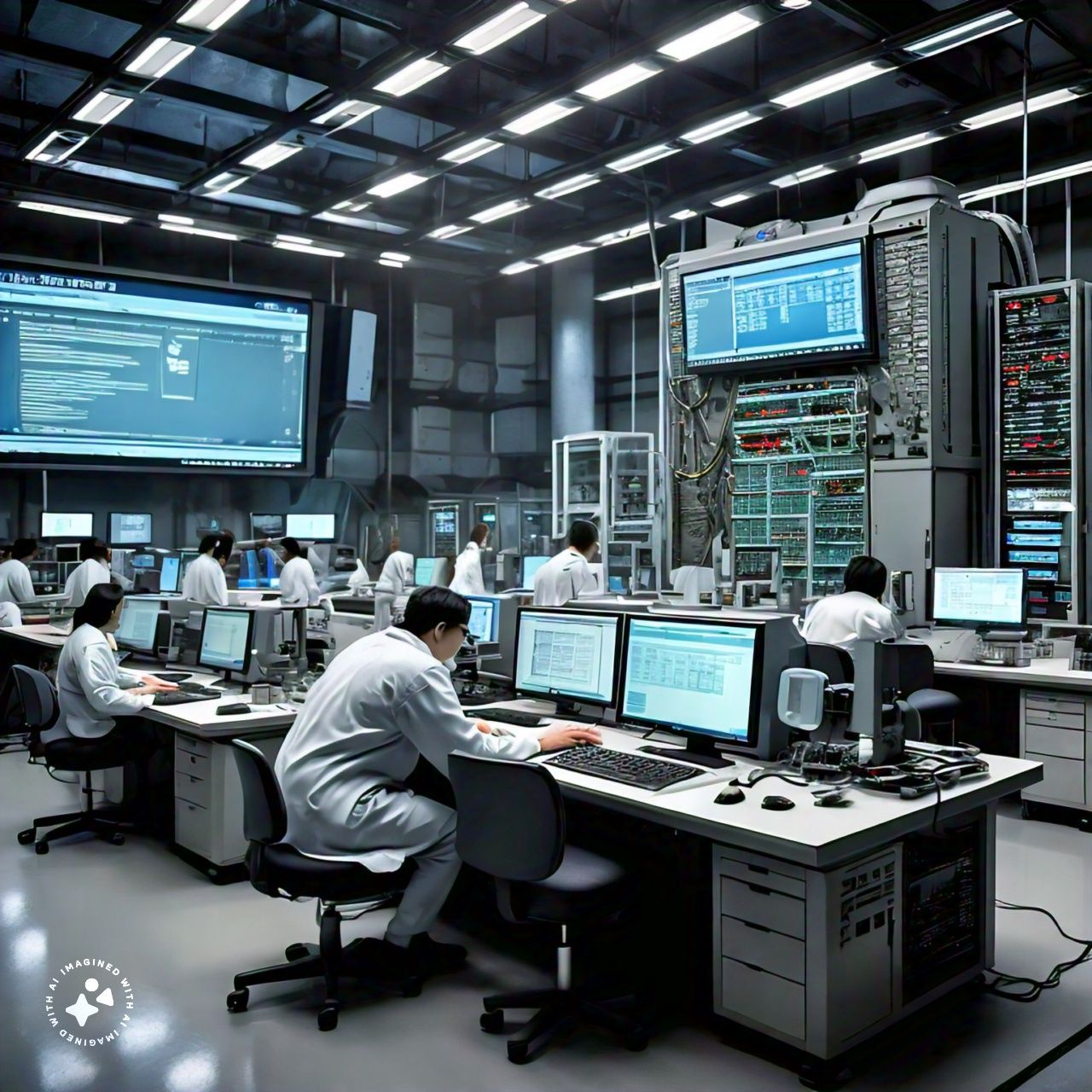
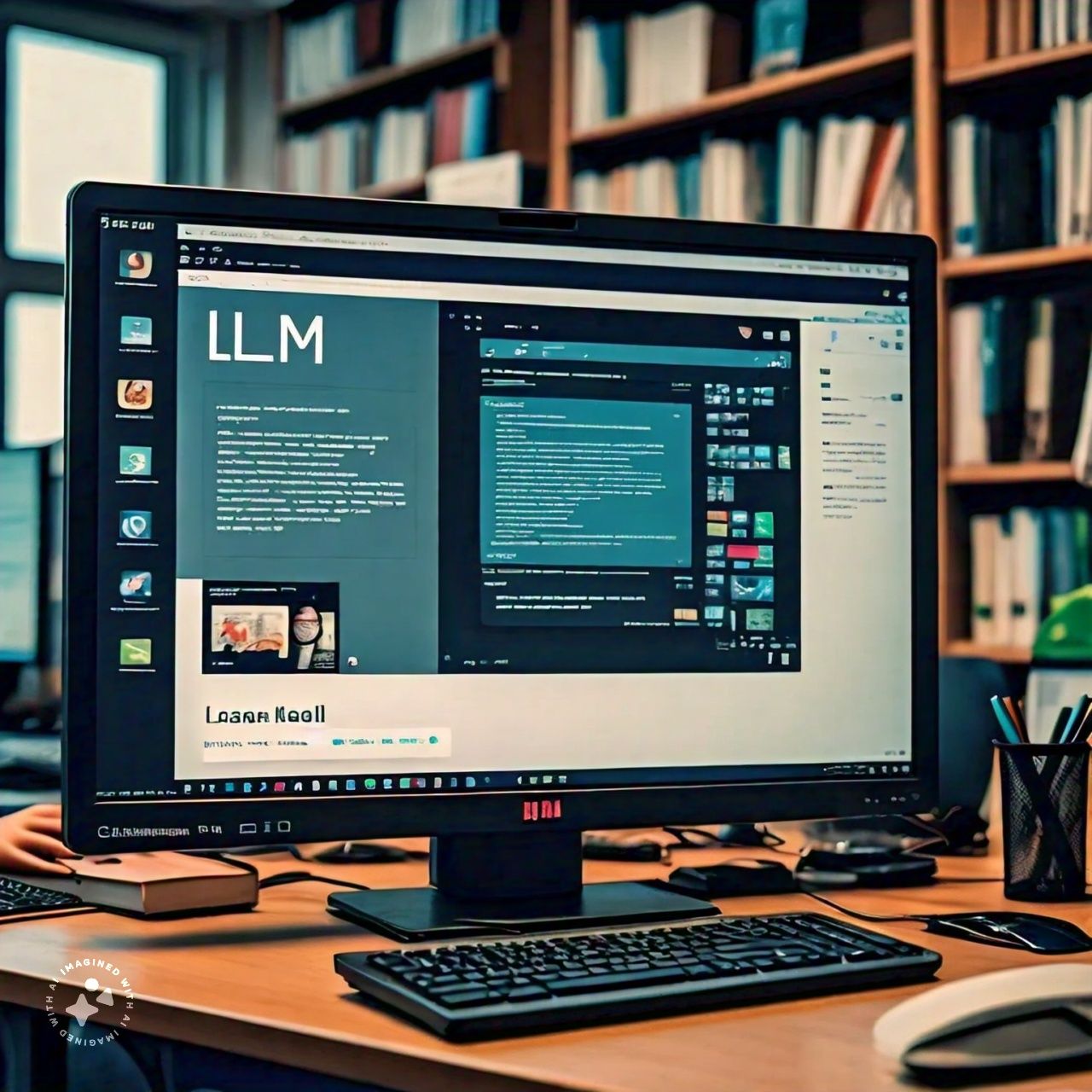
4. Generative AI(GenAI)
Generative artificial intelligence uses the power of large models to do new things, not only regurgitation or provide information on existing things. Learn models and structures and therefore generates something similar but new. You can create things like images, music, text, video, and code.
It can be used to create artwork, write stories, design products, and even help doctors with administrative tasks. But it can also be used by bad actors to create fake news or images that look like photographs but aren’t real, so tech companies are working on ways to.
Generative AI models use complex algorithms to learn patterns within the data they’re trained on and then generate new data that resembles the original. Examples of generative AI include:
1. Image generators (e.g., DeepDream, Prisma)
2. Language models (e.g., myself, ChatGPT)
3. Music generators (e.g., Amper Music, AIVA)
4. Data generators (e.g., synthetic data for medical research)
Generative AI has many potential applications, such as:
1. Art and design
2. Data augmentation
3. Content creation
4. Research and simulation
5. Entertainment
DeepDream is a neural network-based algorithm developed by Google engineer Alexander Mordvintsev and his colleagues in 2015. It’s a type of generative AI that uses a deep learning model to identify and enhance features in images, creating surreal and dreamlike effects.
DeepDream works by:
1. Identifying neural network layers that detect specific features (e.g., edges, shapes, patterns)
2. Amplifying these features to create a “dreamlike” effect
3. Iteratively applying this process to generate increasingly distorted and fantastical images
DeepDream has been used to create striking and imaginative visuals, often resembling psychedelic art or surreal landscapes. It has also inspired new forms of digital art and has applications in areas like:
1. Computer vision
2. Image processing
3. Artistic collaboration
4. Research into neural network dynamics
Some examples of DeepDream’s output include:
– Fantastical creatures and shapes
– Vibrant, swirling patterns
– Dreamlike landscapes and scenery
– Abstract, psychedelic art
DeepDream has captured the imagination of many and has become a iconic representation of the creative potential of generative AI.
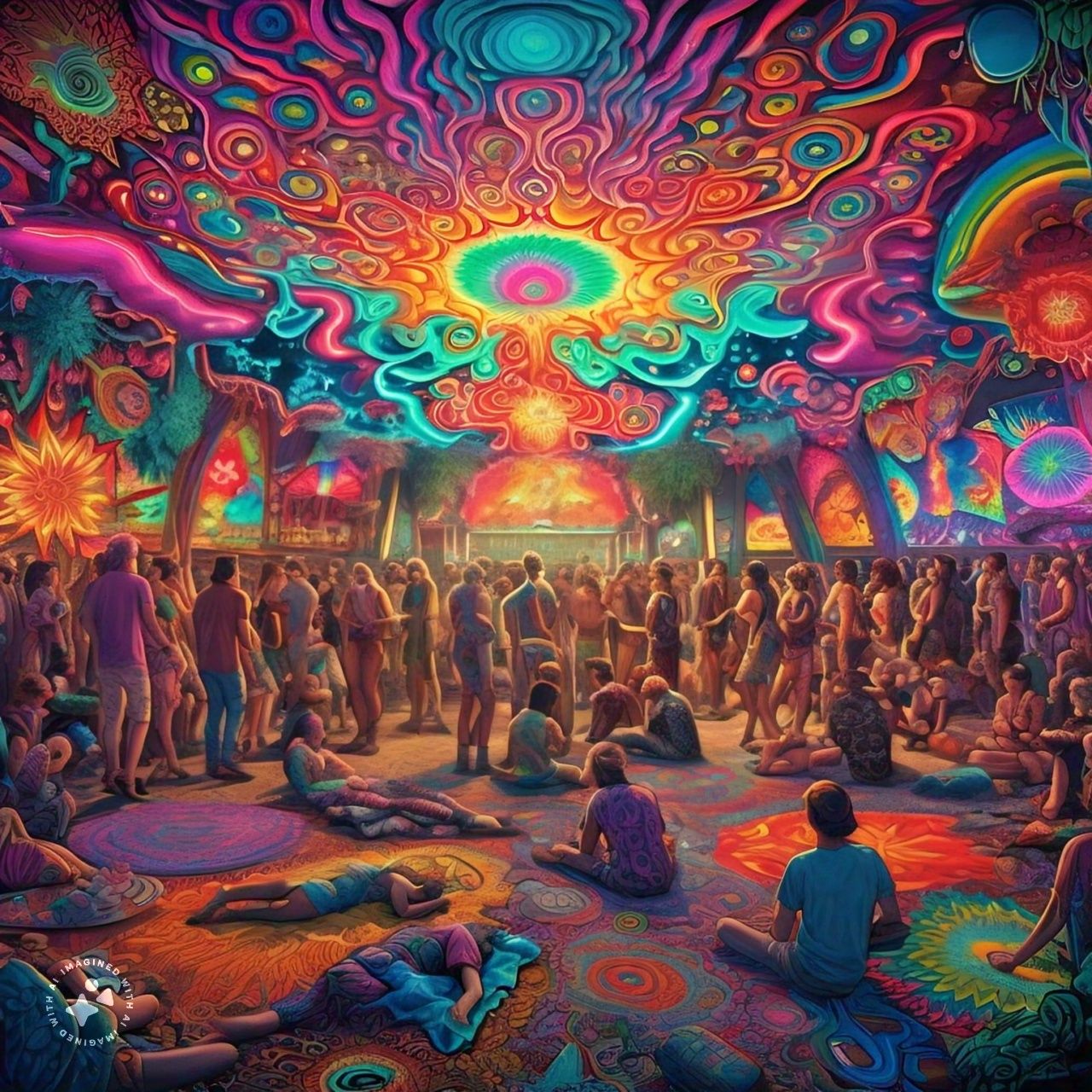
5. Hallucinations
Generative AI systems can create stories, poems, and songs, but sometimes we want the results to be based in truth. Because these systems cannot distinguish between what is real and what is fake, they can provide inaccurate responses that developers call hallucinations or, by the more accurate term, fabrications, just as if someone saw what looks like the outline of a face on the face screen. moon and began to say that there was a real man on the moon.
Developers try to solve these problems by “grounding,” which is providing an AI system with additional information from a trusted source to improve accuracy on a specific topic. Sometimes a system’s predictions are also wrong if a model does not have updated information after being trained.
Hallucinations are sensory experiences that occur in the absence of any external stimulus. They can affect any sensory modality, such as:
1. Visual hallucinations (seeing things that aren’t there)
2. Auditory hallucinations (hearing sounds or voices that aren’t real)
3. Tactile hallucinations (feeling sensations on the skin that aren’t real)
4. Olfactory hallucinations (smelling things that aren’t there)
5. Gustatory hallucinations (tasting things that aren’t there)
Hallucinations can be caused by various factors, including:
1. Neurological disorders (e.g., epilepsy, Parkinson’s disease)
2. Psychiatric conditions (e.g., schizophrenia, bipolar disorder)
3. Sensory deprivation
4. Medications (e.g., hallucinogenic drugs)
5. Sleep and dreaming
6. Neuroscientific experiments (e.g., DeepDream)
Hallucinations can be fascinating and thought-provoking, but they can also be distressing and disruptive to daily life. It’s essential to approach the topic with sensitivity and understanding.
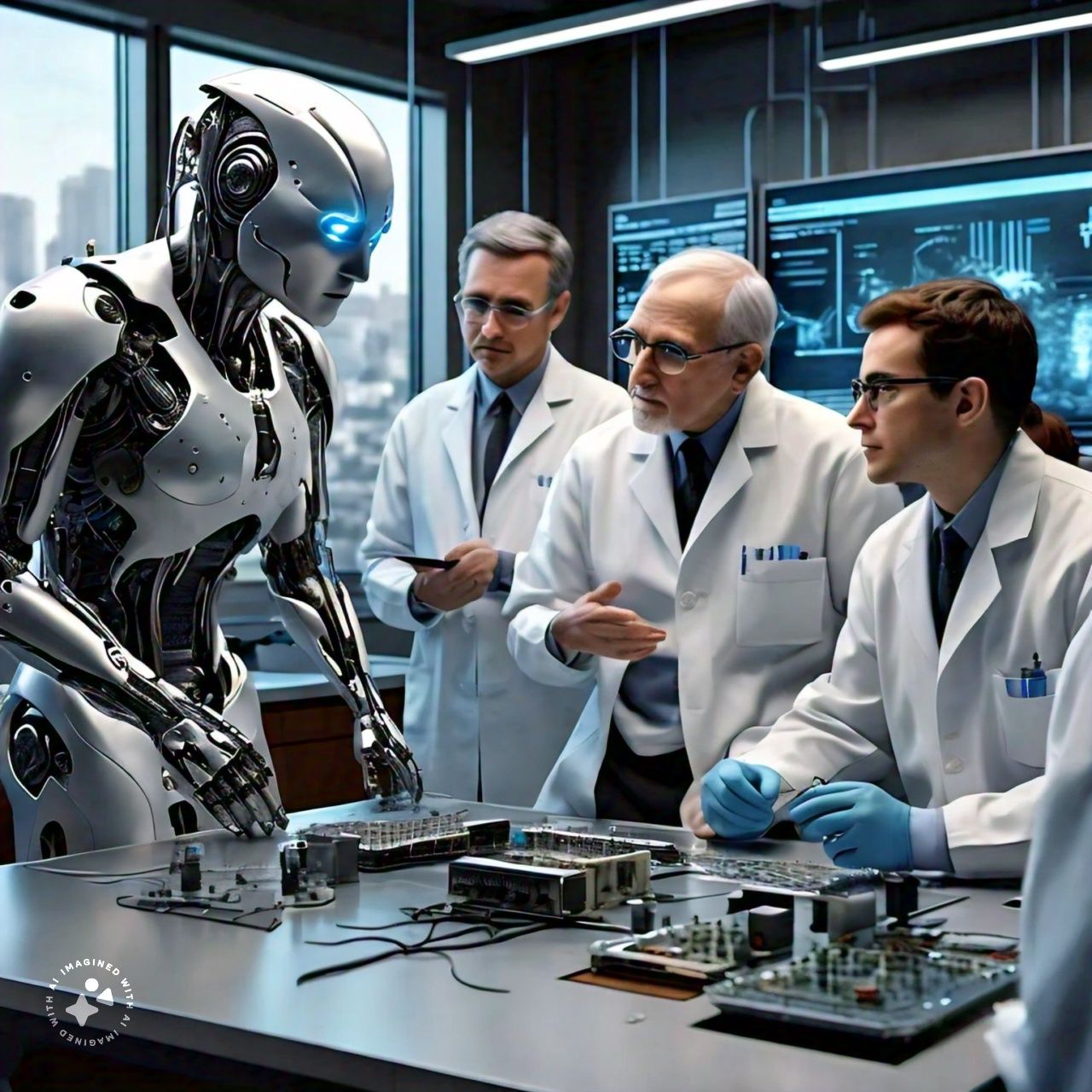
6. Responsible AI
Responsible AI guides people in trying to design safe and fair systems, at all levels, including the machine learning model, the software, the user interface, and the rules and restrictions established for accessing a application.
This is crucial because these systems are often tasked with helping make important decisions about people, such as in education and healthcare, but because they are created by humans and trained with data from an imperfect world, they can reflect any inherent bias. Much of the responsible artificial intelligence implies the understanding of the data used to form the systems and find a way to mitigate any deficiency to help reflect society in general, not just some groups of people.
Responsible AI refers to the development and use of artificial intelligence (AI) in ways that prioritize ethical considerations, transparency, accountability, and human well-being. It involves designing AI systems that are:
1. Inclusive: fair, unbiased, and respectful of diversity
2. Explainable: transparent and interpretable in their decision-making processes
3. Ethical: aligned with human values and moral principles
4. Safe: designed to avoid harm and mitigate potential risks
5. Secure: protected from cyber threats and data breaches
6. Sustainable: environmentally and socially responsible
7. Human-centered: prioritizing human needs and well-being
Responsible AI aims to ensure that AI systems are developed and used in ways that benefit society as a whole, and that their potential risks and challenges are addressed proactively.
Some key aspects of Responsible AI include:
1. Value alignment
2. Risk management
3. Diversity and inclusion
4. Transparency and explainability
5. Human oversight and accountability
6. Continuous testing and evaluation
7. Ethical guidelines and frameworks
By prioritizing Responsible AI, we can promote a future where AI enhances human life without compromising our values or well-being.
7. Multimodal models
A multimodal model can work with different types or modalities of data at the same time. You can look at pictures, listen to sounds, and read words. It’s the best multitasker! You can combine all of this information to do things like answer questions about images.
Multimodal models are AI systems that process and integrate multiple forms of data, such as:
1. Text (e.g., language, captions)
2. Images (e.g., pictures, videos)
3. Audio (e.g., speech, music)
4. Video (e.g., motion, gestures)
5. Sensor data (e.g., temperature, GPS)
These models aim to bridge the modalities, enabling:
1. Cross-modal translation (e.g., image captioning)
2. Multimodal fusion (e.g., combining text and images for sentiment analysis)
3. Multimodal generation (e.g., generating images from text descriptions)
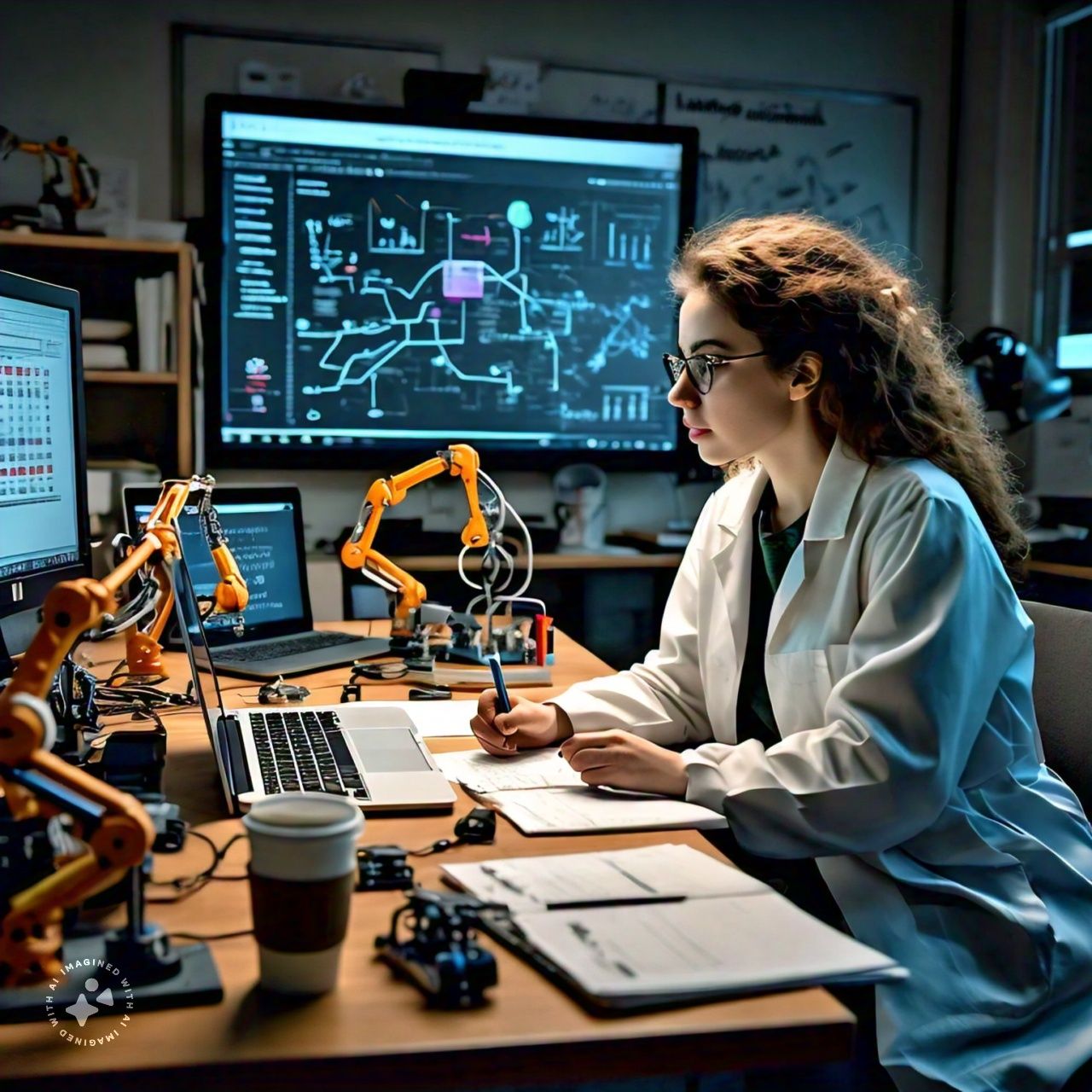
Multimodal models have various applications, including:
1. Virtual assistants
2. Human-computer interaction
3. Sentiment analysis
4. Object detection
5. Language translation
6. Multimodal chatbots
7. Healthcare (e.g., medical image analysis)
8. Robotics (e.g., sensorimotor integration)
Examples of multimodal models include:
1. Transformer-XL
2. Multimodal Transformer
3. Visual BERT
4. Multimodal GPT
5. MM-GPT
These models are advancing rapidly, enabling more sophisticated interactions between humans and machines.
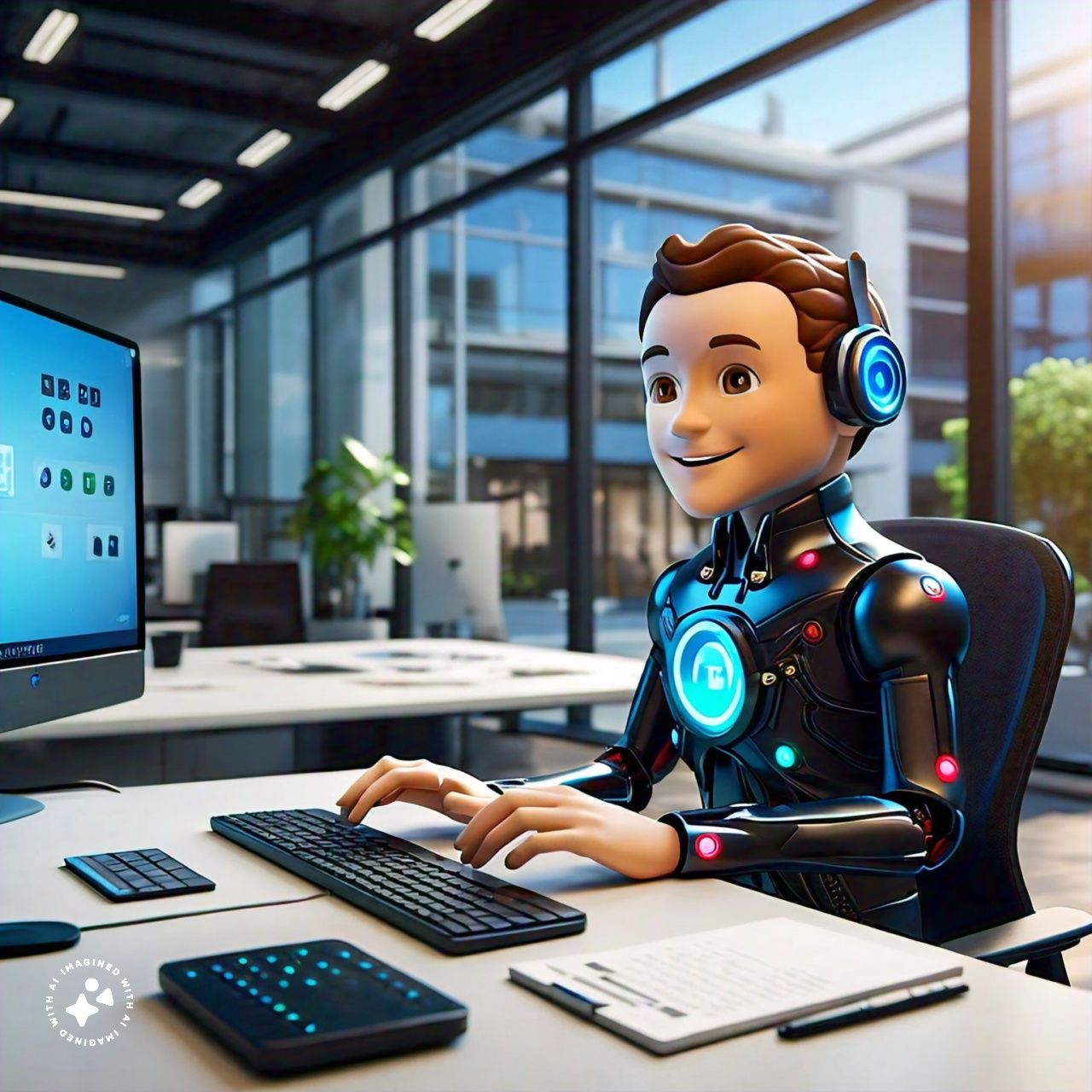
8. Prompts
A PROMPT is an instruction entered into a system in language, images, or code that tells the AI what task to perform. Engineers (and indeed all of us who interact with AI systems) must carefully design clues to get the desired result from large language models. It’s like ordering in a deli: you don’t just order a sandwich, you specify what bread you want, type and quantity of condiments, vegetables, cheeses and meat for a lunch that will be delicious. and nutritious.
A prompt is a cue or a trigger that helps to initiate a response or an action. In the context of AI and language models like myself, a prompt is a piece of input text that guides me to generate a response. It can be a question, a statement, a phrase, or even just a few words.
Prompts can be used to:
– Ask a question
– Provide context for a conversation
– Give instructions or tasks
– Offer ideas or topics to explore
– Elicit creative writing or responses
Examples of prompts include:
– “What is the meaning of life?”
– “Write a story about a character who…”
– “Summarize this article for me.”
– “Generate a poem about nature.”
– “Tell me a joke.”
In essence, prompts help to get the conversation started and guide the direction of the response. It’s a word, phrase, or question that helps generate an idea or a response.
9. Copilots
A co-pilot is like a personal assistant who works alongside you on all types of digital applications, helping you with tasks like typing, coding, summarizing, and searching. She can also help you make decisions and understand a lot of data.
The recent development of large language models has made co-pilots possible, allowing them to understand natural human language and provide responses, create content, or take actions while working with different computer programs.
Co-pilots are equipped with responsible AI protections to ensure they are safe and used correctly. Like a co-pilot on a plane, it’s not in charge (you are), but it’s a tool that can help you be more productive and efficient.
Copilots are AI tools that assist and augment human capabilities, much like a copilot in an aircraft. In the context of generative AI, copilots can help guide the AI system to produce more accurate, relevant, and ethical outputs. They can also help humans to better understand and trust AI decision-making processes.
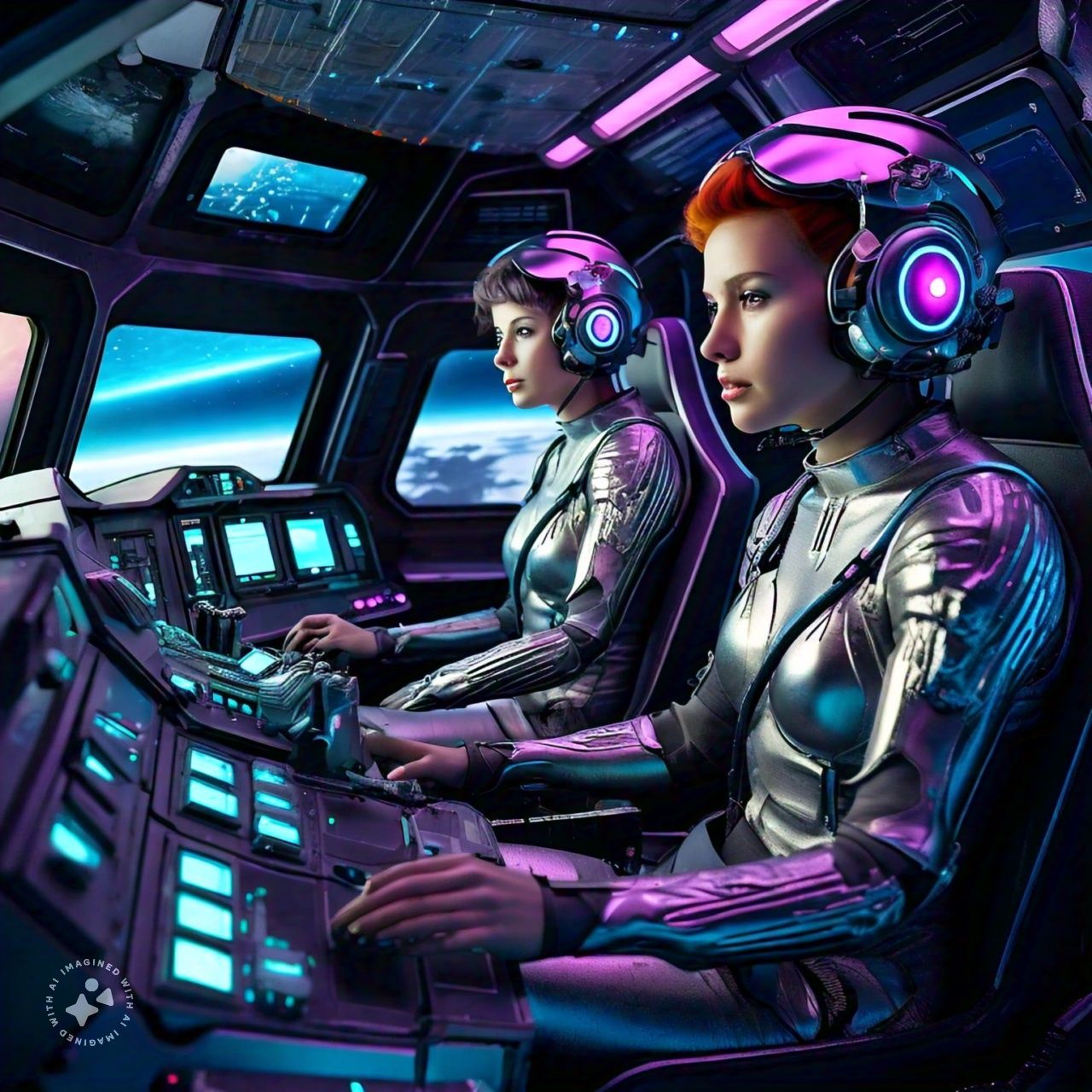
In essence, copilots are designed to:
1. Enhance productivity and efficiency
2. Improve accuracy and relevance
3. Provide explainability and transparency
4. Ensure accountability and responsibility
Copilots can be applied in various domains, such as:
1. Writing and content creation
2. Data analysis and science
3. Art and design
4. Decision-making and strategy
By leveraging copilots, we can harness the power of AI while maintaining human oversight, creativity, and values.
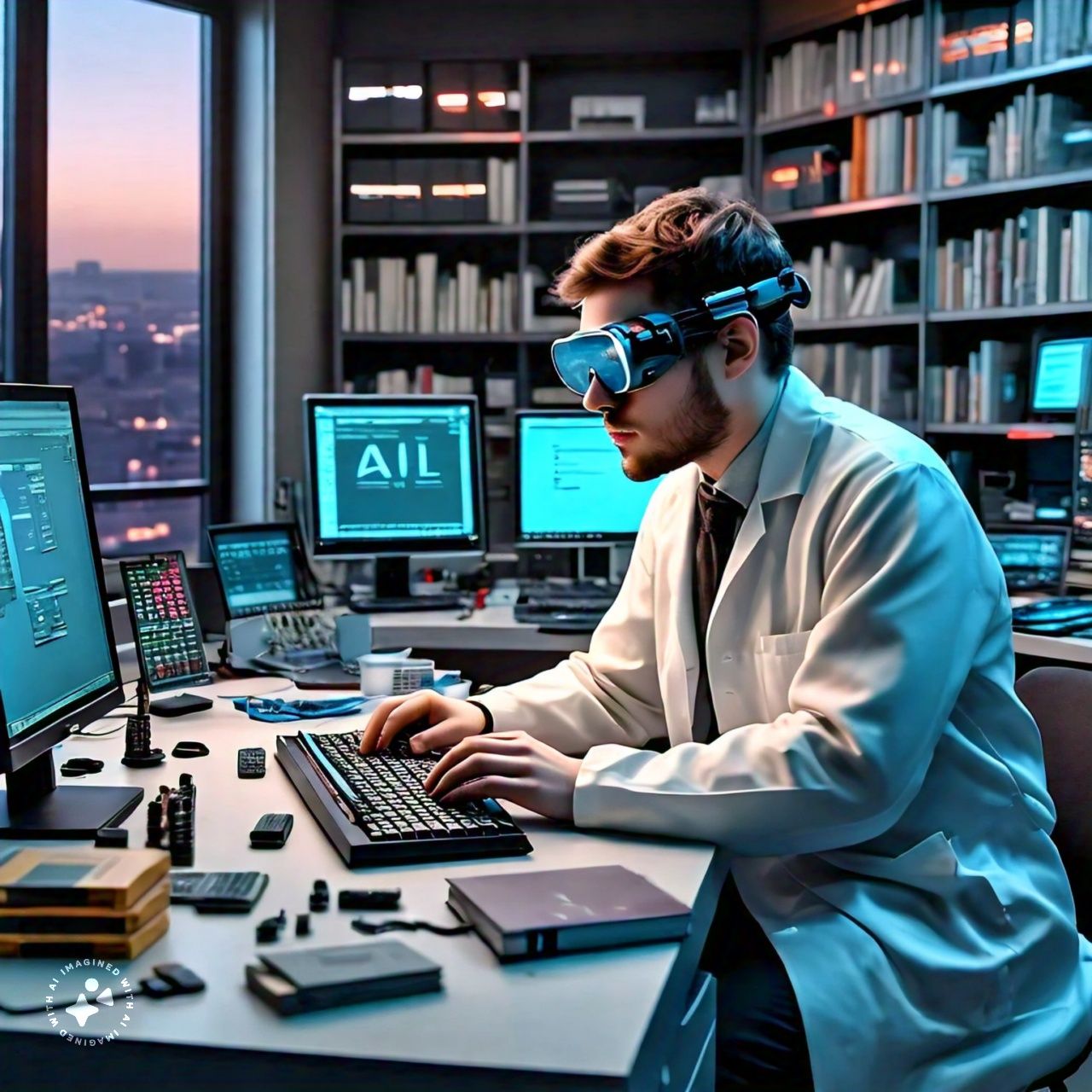
10. Plugins
Plugins are a bit like adding apps to your smartphone: they step in to meet specific needs that may arise, allowing AI apps to do more without having to modify the underlying model.
They are what allow co-pilots to interact with other software and services, for example. They can help AI systems access new information, perform complicated mathematical operations, or communicate with other programs. They make AI systems more powerful by connecting them to the rest of the digital world.
Plugins and AI applications can intersect in various ways, enabling innovative and powerful capabilities. Here are some examples:
1. AI-powered plugins for image editing software, like Adobe Photoshop, that utilize machine learning algorithms for image enhancement, segmentation, and manipulation.
2. Natural Language Processing (NLP) plugins for chatbots and virtual assistants, enabling more accurate language understanding and generation.
3. Predictive analytics plugins for business intelligence tools, leveraging machine learning to forecast sales, customer behavior, and market trends.
4. AI-driven plugins for content generation, such as automated writing, video creation, and music composition.
5. Plugins that integrate AI-powered chatbots into websites and applications, enhancing customer support and engagement.
6. AI-based plugins for fraud detection and security enhancements in e-commerce and financial applications.
7. Plugins that utilize AI for personalized recommendations in e-commerce, music, and video streaming services.
8. AI-powered plugins for sentiment analysis and social media monitoring in marketing and analytics tools.
These integrations demonstrate how plugins and AI can combine to create more intelligent, efficient, and innovative applications, transforming various industries and workflows